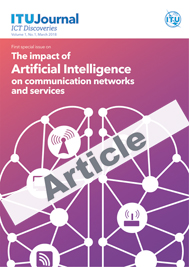 | The rapid growth of civilian vehicles has stimulated the development of advanced driver assistance systems (ADASs) to be equipped in-car. Real-time autonomous vision (RTAV) is an essential part of the overall system, and the emergence of deep learning methods has greatly improved the system quality, which also requires the processor to offer a computing speed of tera operations per second (TOPS) and a power consumption of no more than 30 W with programmability. This article gives an overview of the trends of RTAV algorithms and different hardware solutions, and proposes a development route for the reconfigurable RTAV accelerator. We propose our field programmable gate array (FPGA) based system Aristotle, together with an all-stack software-hardware co design workflow including compression, compilation, and customized hardware architecture. Evaluation shows that our FPGA system can realize real-time processing on modern RTAV algorithms with a higher efficiency than peer CPU and GPU platforms. Our outlook based on the ASIC-based system design and the ongoing implementation of next generation memory would target a 100 TOPS performance with around 20 W power. |
|